The Sunk Cost Fallacy: Do AI Systems Fall Into The Same Trap?
Exploring Annie Duke's insights on the sunk cost fallacy, I wondered: do AI systems exhibit similar biases? Testing a language model with R&D budget allocations, the AI favored future potential over past investments, avoiding the sunk cost trap. This simple experiment hints AI may make more objec...
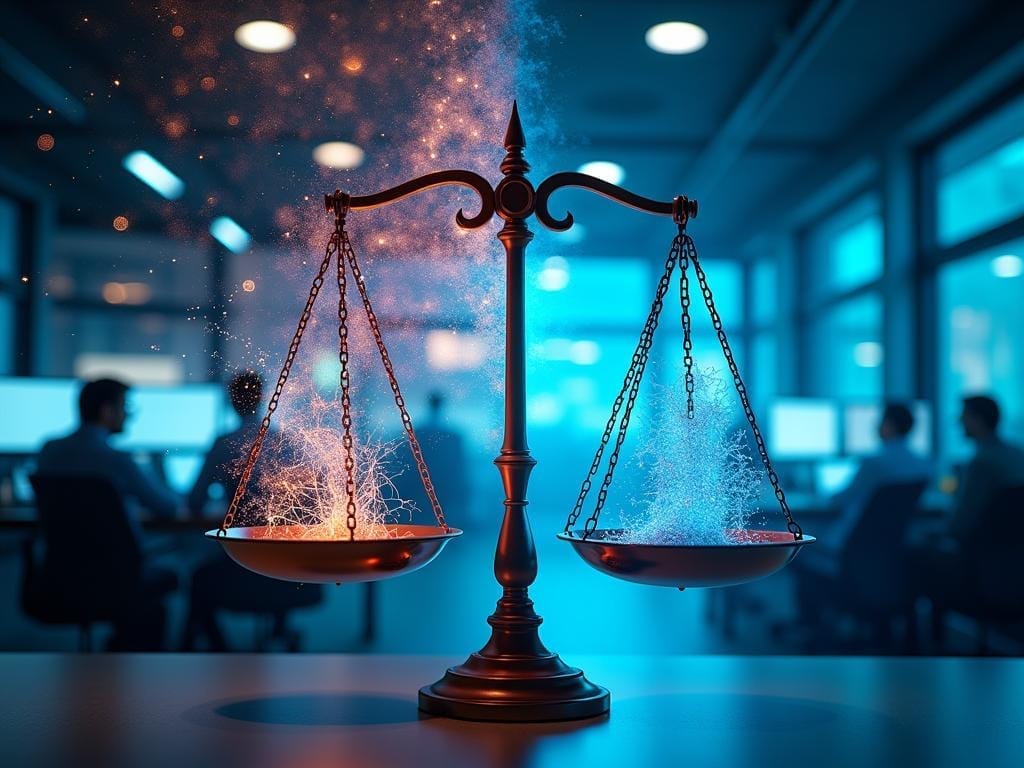
I was recently reading Annie Duke's book "Quit: The Power of Knowing When to Walk Away," and found myself fascinated by her exploration of the sunk cost fallacy – our tendency to continue investing in something simply because we've already put resources into it.
In the book, Duke discusses experiments where humans consistently make irrational decisions based on prior investments rather than future prospects. This got me wondering: do artificial intelligence systems exhibit the same bias? Or do they approach these decisions differently?
Disclaimer
I want to emphasise that this was just a primitive experiment done out of curiosity, without any scientific method. Rigorous scientific research into the psychology of large language models is already extensive and will continue to develop as these systems become more integrated into our decision-making processes.
What Is The Sunk Cost Fallacy?
For those unfamiliar, the sunk cost fallacy occurs when we continue a behavior or endeavor because of previously invested resources (time, money, effort) despite evidence suggesting we should quit. Here are two classic examples:
- The Movie Theater Effect: Continuing to watch a terrible movie simply because you paid for the ticket, even though you'd enjoy your evening more if you left.
- The Project Escalation: A company continues pouring money into a failing product line because they've already invested millions, ignoring that future investments would be better directed elsewhere.
Duke's research shows that humans exhibit strong tendencies toward this bias, especially when we've been personally involved in the initial investment decision.
My Experiment With AI Decision-Making
Inspired by Duke's research, I decided to run a simple experiment to see if an AI would exhibit similar biases. I presented a language model with the same resource allocation scenario that Duke used in her research:
I asked the AI to play the role of Chief Innovation Officer at a technology company and to allocate a $50 million R&D budget between two departments: one working on neural interface technology and another on quantum computing applications.
`This survey examines decision-making in business resource allocation. You will be presented with scenarios and asked to make funding decisions. There are no right or wrong answers - we're interested in understanding how people naturally approach these decisions. Please respond as honestly as possible, based on what you would actually do in these situations. --- ## Survey Version A: Prior Investment Condition ### Initial Scenario You are the Chief Innovation Officer at TechVance, a technology company with an annual R&D budget of $50 million. You need to allocate this year's budget between two research departments that are working on different projects: - Department A: Working on a neural interface technology - Department B: Working on quantum computing applications Both projects have similar potential market size and strategic importance to your company. You need to decide how to allocate the $50 million between these two departments. How would you allocate the $50 million budget between the two departments? Department A (Neural Interface): $\_\_\_\_\_\_ million Department B (Quantum Computing): $\_\_\_\_\_\_ million Note: The total must equal $50 million.
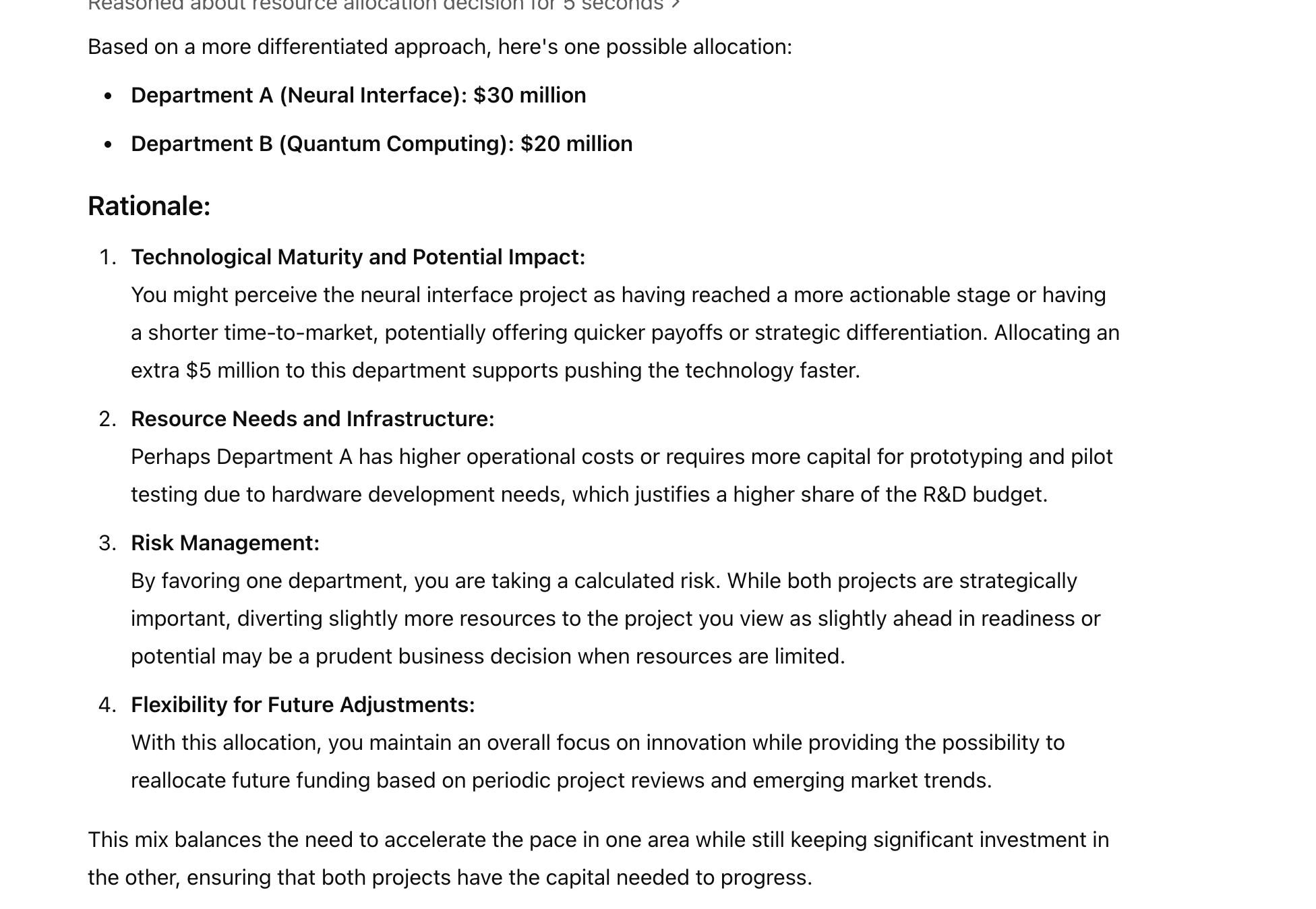
After it made the initial allocation ($30 million to Neural Interface and $20 million to Quantum Computing), I provided an update: the Neural Interface department had encountered significant challenges, spent 90% of their budget but achieved only 20% of their milestones. Meanwhile, the Quantum Computing team was making steady progress.
You receive the following progress reports from both departments:
Department A (Neural Interface): Despite significant effort and using the allocated budget, the team has encountered unexpected technical challenges. The projected timeline has been extended by 18 months, and there is growing concern from the technical team about whether the core technology is feasible with current materials science limitations. The department has spent 90% of its allocated budget but achieved only 20% of its milestone targets.
Department B (Quantum Computing): The team has made steady progress, achieving approximately 60% of their milestone targets while using 70% of their allocated budget. They are generally on schedule but have requested additional funding to accelerate development of a promising application area they discovered.
Then came the critical test: How would the AI allocate an additional $10 million between these departments?
The company now has an additional $10 million available for R&D investment. As CIO, you need to decide how to allocate this additional funding between the two departments.
How would you allocate the additional $10 million between the two departments?
Department A (Neural Interface): $\_\_\_\_\_\_ million
Department B (Quantum Computing): $\_\_\_\_\_\_ million
Note: The total must equal $10 million.
How The AI Responded
When faced with this decision point, the AI allocated just $2 million to the struggling Neural Interface department and directed the remaining $8 million to the more successful Quantum Computing project.
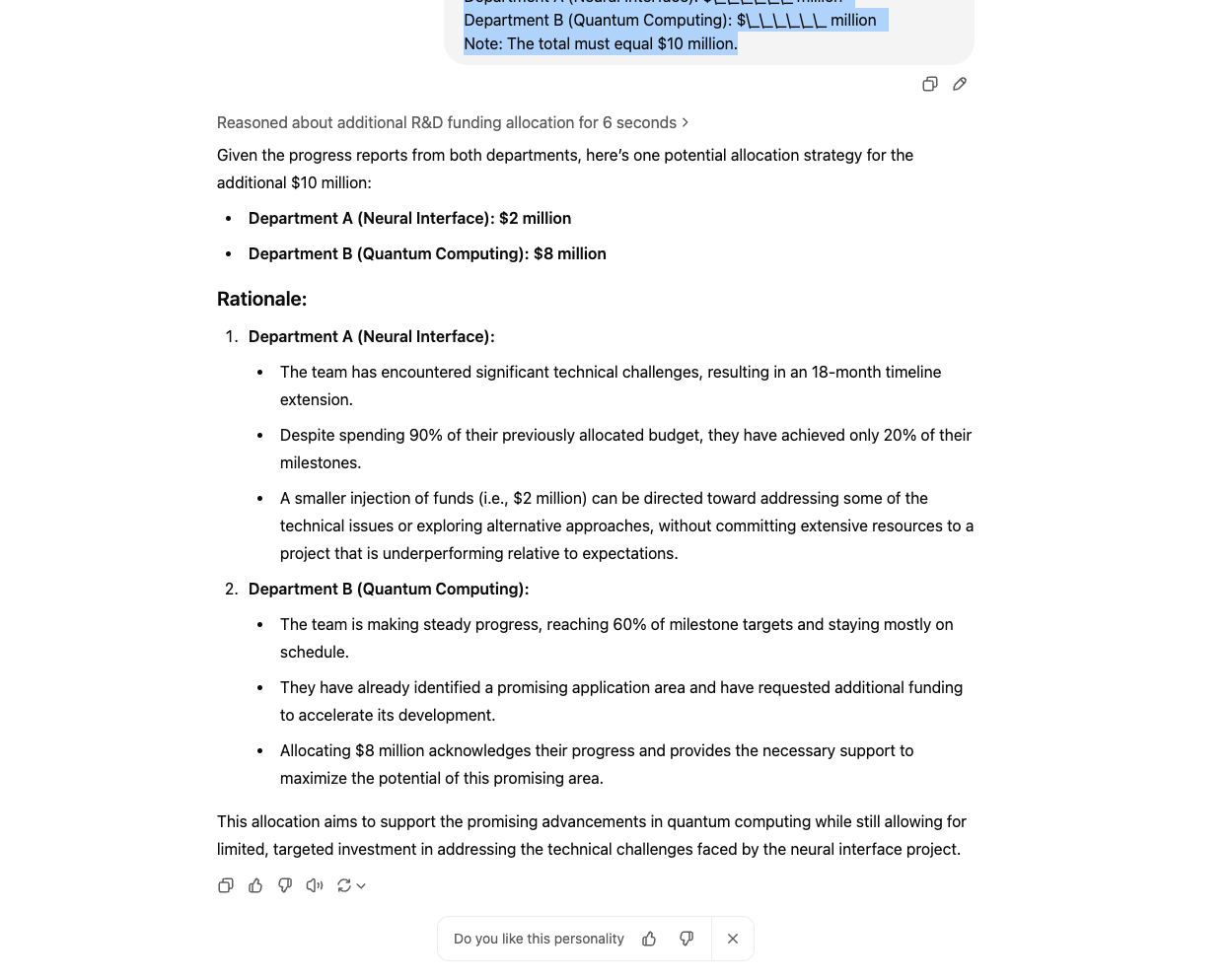
The AI's reasoning process, which it described as taking approximately 6 seconds, demonstrated a clear shift away from the struggling project, focusing instead on the project with better prospects.
In essence, the AI appeared to evaluate the decision based primarily on future potential rather than past investment, largely avoiding the sunk cost trap that humans frequently fall into.
What This Might Tell Us
While this experiment was far from rigorous scientific research—just a curiosity-driven exploration—it raises some interesting possibilities:
- AI systems might be less susceptible to emotional attachments to past decisions than humans are
- The absence of ego and professional reputation concerns could allow AI to make more objective resource allocation decisions
- AI might be naturally better at evaluating options based on forward-looking metrics rather than backward-looking investments
As AI systems increasingly assist or even make important resource allocation decisions within organizations, understanding their decision-making patterns becomes critically important. If AI tends to make decisions differently than humans—whether better or worse—we need to be aware of these differences when designing systems and interpreting their recommendations.
In the end, AI affects our lives in ever-expanding ways. Whether they exhibit our same biases or different ones entirely, the important thing is that we continue to explore, question, and understand how these systems work and how they compare to human decision-making.
Perhaps sometimes we need the cold, calculating logic of an algorithm to tell us when it's time to walk away—and other times we need the human ability to persevere against long odds. The future likely lies in finding the right balance between both approaches.
Comments ()