The insane waste of time and money in LLM token generation
Navigating the complexities of editing content generated by AI can be a challenge, particularly as documents grow larger. The Pareto Principle highlights how finishing touches often become more tedious than the initial creation. This post explores common pitfalls in modifying generated content an...
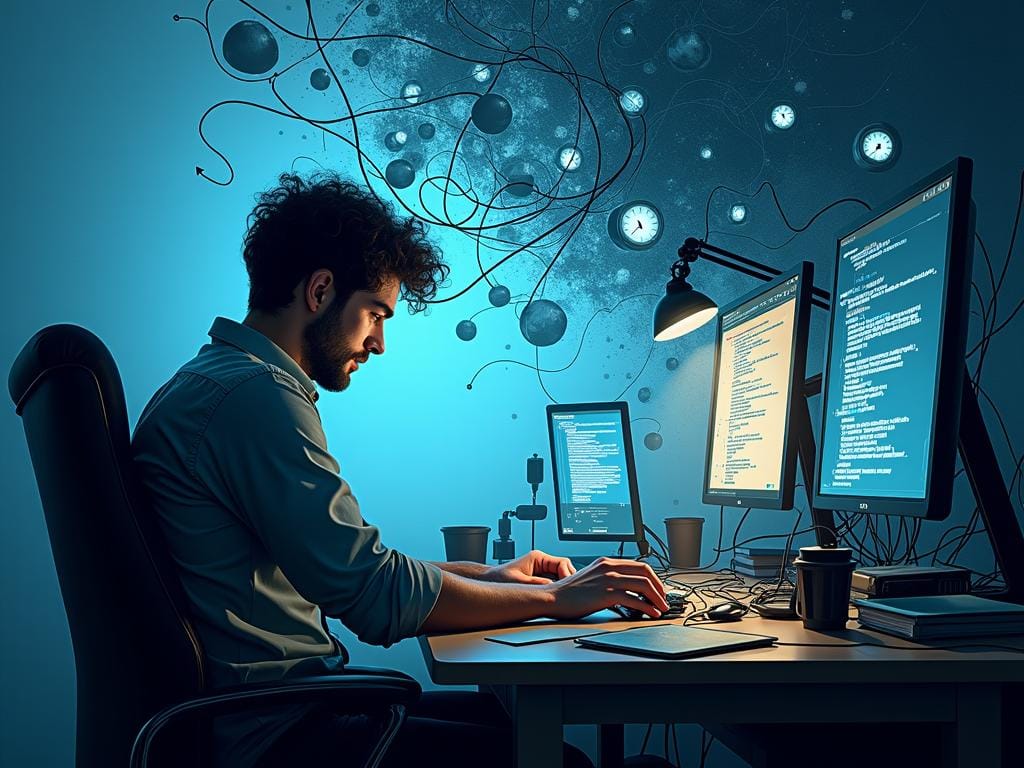
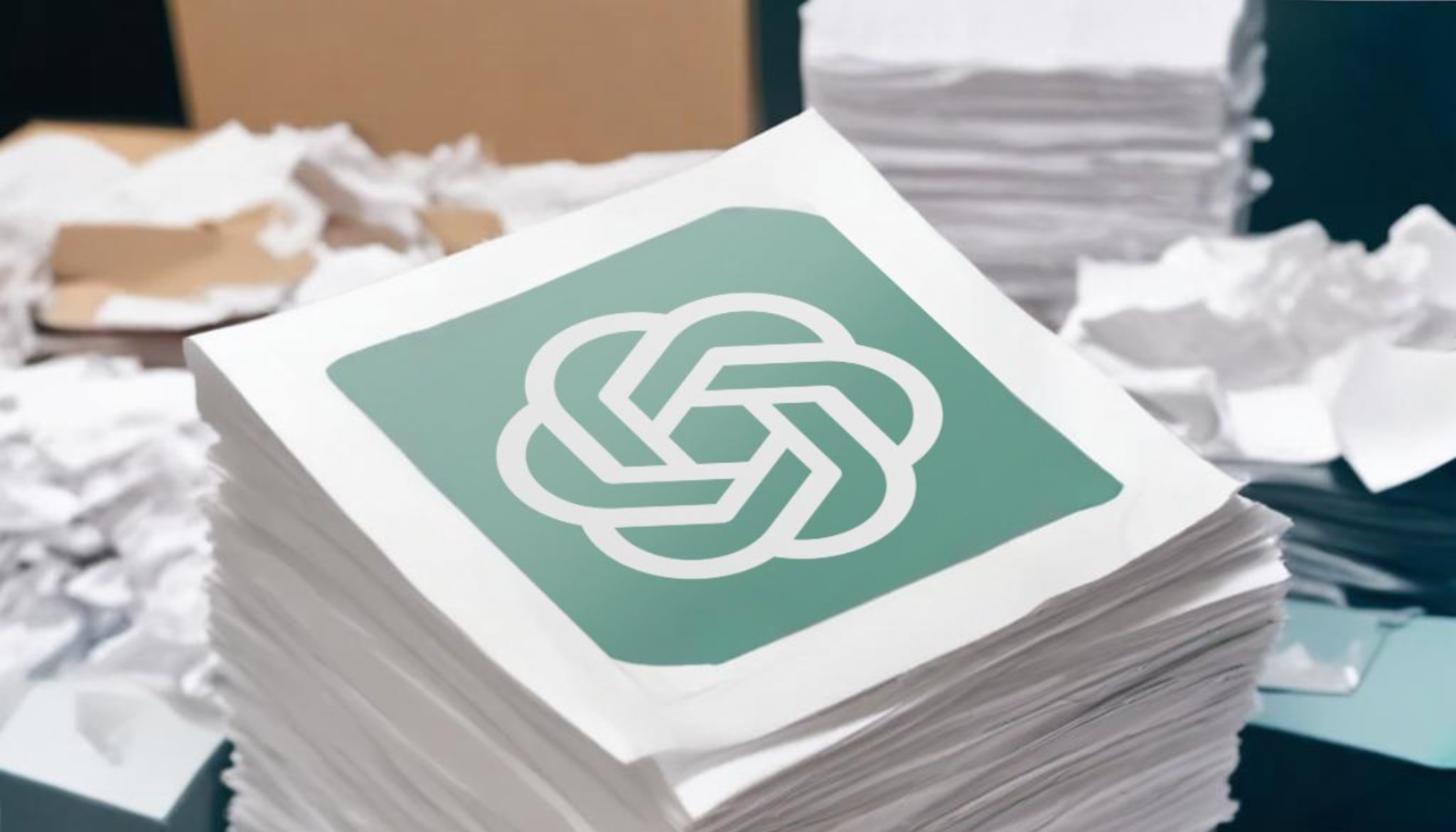
If you’ve worked with GPT and other LLMs like Claude or Mistral, you’ve likely noticed a big boost in productivity. However, the more you use Generative AI, the more you encounter the Pareto Principle — where finishing touches become harder than creating the main piece.
This issue is more noticeable as your content (like code or text) grows, making even small changes feel tedious, inefficient, and costly in terms of time (Delays) and resources. I’ve studied this pattern for years, especially in code generation, and I want to share my observations and potential solutions to these challenges, helping you create great work with a lot less cost, effort and delays.
Sounds abstract? Let’s take an example.
I need to create a landing page for my new product/service. The landing page should be designed to attract and convert visitors by clearly communicating the value proposition, features, and benefits.....
You inspect it and like it, but then you want this tiny change.
So, you ask GPT to make the modification.
... the hero section should ...
A few seconds (or minutes) later, depending on the length of the original document, it regenerates the entire document.
It does some changes, but it's not what you expect.
So, you ask again.
... make some adjustments. The hero section is too cluttered.
A few seconds (or minutes) later, depending on the length of the modified document...And again, it regenerates the entire document.
It's better, but still not what you expected.
And on and on.
Let's illustrate this behaviour (Thanks Claude:)
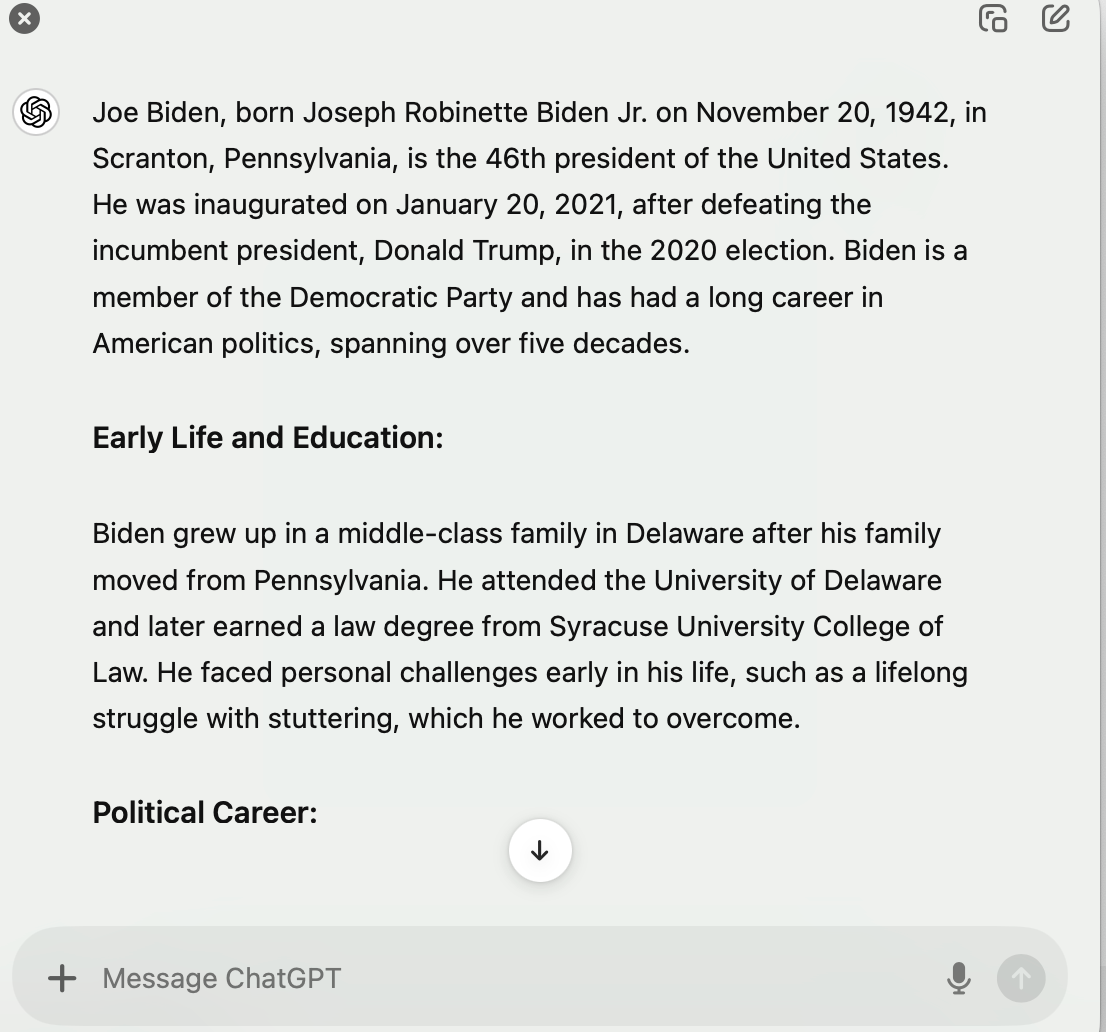
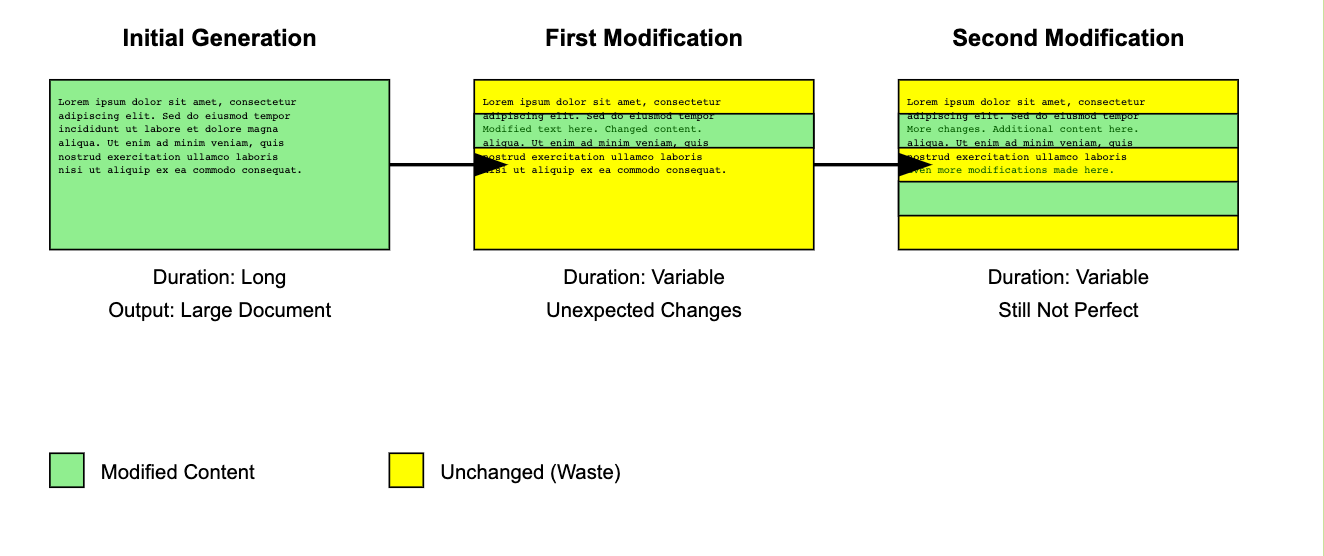
The larger the document, the more tedious, time-consuming, and expensive this undertaking becomes.
It can be very frustrating, especially after having a lot of hope and feeling enthusiastic about the initial generation.
We encounter these behaviors and challenges across a lot of use cases:
Let's take a quick look at some examples (very brief)
- Summarising: Big context and a summary in one piece.
☞ Works well if you have a good prompt and a good model - Answering questions on a long document
☞ Works pretty well, even for long documents, especially if you have caching. - Initial code generation (greenfield) for say, a hello world application
☞ Usually easy and quick if the model is good. - Modifying generated code (after initial generation)
☞ Depends heavily on the reasoning capabilities of the underlying model, the size of the files, and the change you want to make. - Editing existing documents, contracts, etc.
☞ Depends on the number of changes, the length of those changes and how they are distributed (in one paragraph or over many paragraphs etc.)
In look at it in a more abstract way:
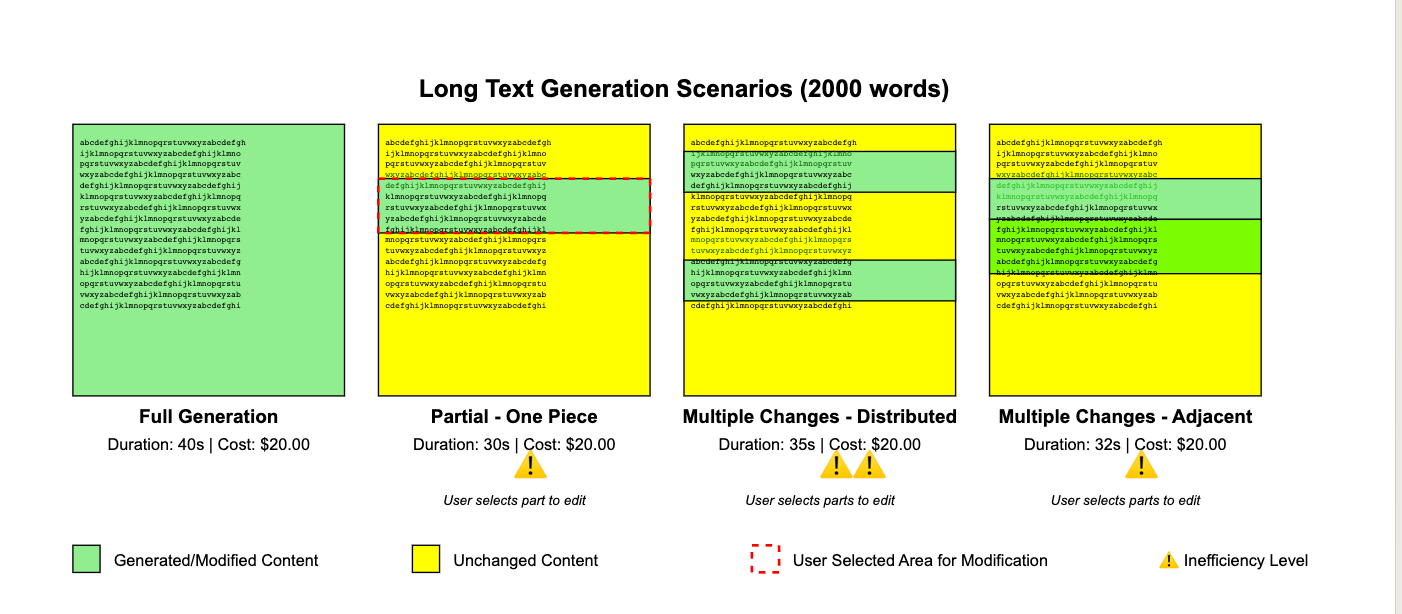
If we look at these scenarios from 1000 feet up, we'll find some patterns, or let's say scenarios (high level).
Initial generation of long text
Duration and number of tokens depend heavily on the length of the text, the speed and the cost of the model. Progress is being made in this area almost daily across the industry.
Editing parts of a long text that are close together:
In many editors, such as Canvas or Artifacts, you can select parts of the area you want to edit, reducing the amount of change and avoiding generating a lot of unmodified text.
Editing several parts of a short text
When we are dealing with short text, it does not really matter whether the change is distributed or not, the whole generation does not take that much time, so this should not be an issue for most fast and cheap models.
Editing multiple distributed parts of a long text
This is the most difficult part. If you want to make changes that affect several places in the text. You could of course select each section and apply the change, but this can be very inefficient, time consuming and error prone. Alternatively, you can re-generate the whole text, but this too can be fraught with difficulties and problems:
- Code Repetition:Some Language Learning Models (LLMs) unnecessarily repeat entire codebases when only a difference (diff) is needed.
- Accidental Errors:Inserting code can result in unintended deletions or the introduction of bugs.
- Waiting time: This is probably the most painful experience for any developer or person trying to "chat" with a long document.
- Limited Length of Generated Code:Many LLMs, when asked to generate very lengthy documents of say +/- 2000 tokens, will start outputting placeholders (implicitly asking you to fill them with the previous context). Even the great models like Claude-Dev, with amazing reasoning capabilities, sometimes do that—though admittedly not that often if you have a well-structured codebase.
- High Costs of Output Tokens:Significant usage of output tokens leads to increased costs, making large-scale modifications economically unfeasible.
- And probably many more ...
How can you avoid some of these problems?
- Versioning and Rollback:Traditional version control systems help, but they don't solve the inefficiency in the modification process itself.
- Make Your Document Smaller:So the LLM can select, with reasoning, which parts to read and process (and modify). From a software engineering perspective, this is the best practice anyway, even without LLMs.
- Maybe you have some other tricks for dealing with this problem?
But the industry as a whole is also evolving
LLMs have solved a lot of limitations in reading documents. The context of LLMs has become incredibly large—up to 10 million tokens for some models on the market. This makes the analysis of very large documents possible like never before.
This is great for tasks like question answering, especially with context caching mechanisms—it's a game changer.
Also, with the speed of inference, it takes less time to analyze documents and generate content (with moderate length, up to +/- 2000 tokens, unless you use a fine-tuned model trained to process more).
But the problem of changing (not reading!) large documents across documents remains a major challenge.
In my next blog post, we'll talk about ways to overcome these problems, using real-world examples.
Comments ()